INTRODUCTION
Independent component analysis (ICA) is the most commonly used computational tool for identifying and characterizing underlying brain functional networks. One of the challenging research topics in ICA is how to perform group ICA for multi-subject imaging studies. Our research on group ICA methodology focused on development of probabilistic group ICA framework for estimating brain functional networks based on multi-subject fMRI data. We have proposed general temporal-concatenation group ICA (TC-GICA) models that can accommodate different types of between-subject variability in temporal responses and model wide variety of neural signals under different experimental tasks (Guo and Pagnoni, 2008; Guo, Biometrics, 2011). In addition to TC-GICA, we have developed a novel hierarchical group ICA method to formally model subject-specific effects in both temporal and spatial domains in fMRI data (Guo and Tang, 2013). Furthermore, we have recently developed a hierarchical covariate-adjusted ICA (hc-ICA) model that provides a formal statistical framework for estimating covariate effects and testing differences between brain functional networks. Our method provides a more reliable and powerful statistical tool for evaluating group differences in brain functional networks while appropriately controlling for potential confounding factors. We plan to release a matlab toolbox for hc-ICA in 2016.
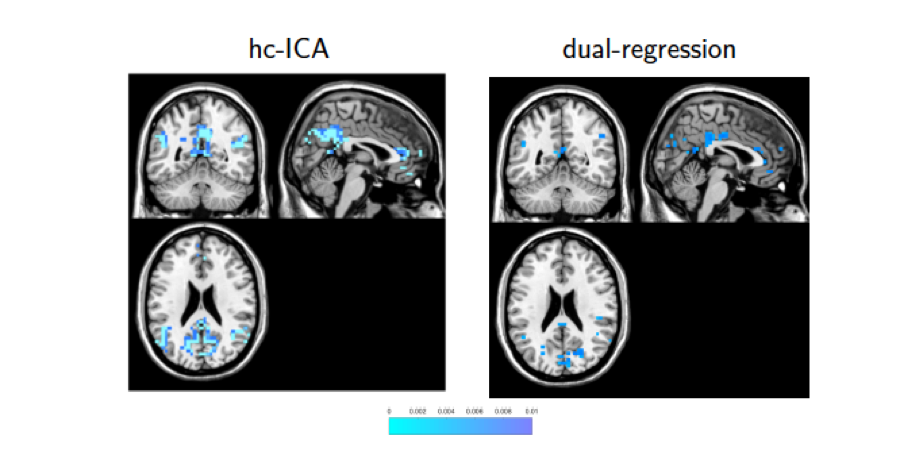
REFERENCES
Shi, R and Guo, Y. (2016+). Investigating Differences in Brain Functional Networks Using a Hierarchical Covariate-adjusted ICA Model. Annals of Applied Statistics. Accepted.
Guo, Y and Tang, Li. (2013). A hierarchical probabilistic model for group independent component analysis in fMRI studies, Biometrics, doi: 10.1111/biom.12068.
Guo Y (2011). A general probabilistic model for group independent component analysis and its estimation methods. Biometrics. 67(4): 1532-1542.
Guo Y and Pagnoni G (2008). A unified framework for group independent component analysis for multi-subject fMRI data. NeuroImage 42: 1078-1093. Listed in ScienceDirectðs Top 25 hottest articles of NeuroImage between July-Sep. 2008.
Guo Y (2008). Group Independent Component Analysis of Multi-subject fMRI data: Connections and Distinctions between Two Methods. IEEE Proceedings of the 2008 International Conference on BioMedical Engineering and Informatics v2: 748-752.